The banner ads on your browser, the route Google maps suggests for you, the song Spotify plays next: algorithms are inescapable in our daily lives.
Some of us are already aware of the mechanisms behind a targeted ad or a dating profile that lights up our phone screen. However, few of us may actually stop to consider how this technology plays out in the hiring sector. As with any major technological advancement, it usually takes society (and legislation) a while to catch up and adjust for unintended consequences. Ultimately, algorithms are powerful tools. Like any tool, they have the potential for societal benefit or harm, depending on how they’re wielded.
Here to weigh in on the matter is Assistant Professor of Information Systems & Operations Management Prasanna Parasurama, who recently joined Emory Goizueta Business School’s faculty in fall of 2023.
This interview has been edited for clarity.
Describe your research interests in six words.
Six words…that’s difficult to do on the spot. How about “the impact of AI and other digital technologies on hiring.” Is that condensed enough?
That works!
What first interested you in the intersection of AI and hiring practices?
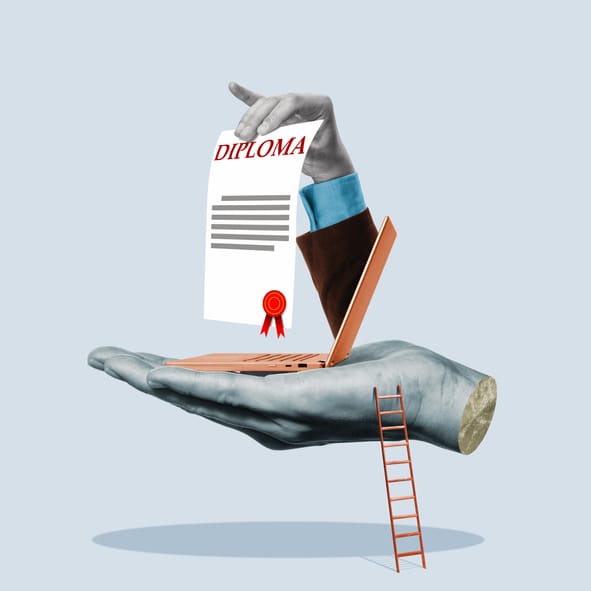
Before I did my PhD, I was working as a data scientist in the HR analytics space at a start-up company. That is where my interest in the topic began. But this was a long time ago. People hadn’t started talking much about AI, or algorithmic hiring. The conversation around algorithmic bias and algorithmic fairness picked up steam in the second or third year of my PhD. That had a strong influence on my dissertation focus. And naturally, one of the contexts in which both these matters have large repercussions is in the hiring space.
What demographics does your research focus on (gender identity, race/ethnicity, socioeconomic status, all of the above)? Do you focus on a particular job sector?
My research mostly looks at gender and race for two main reasons. First, prior research has typically looked at race and gender, which gives us a better foundation to build on.
Second, it’s much easier to measure gender and race based on the data that we have available—from resumes, from hiring data, like what we collect from the Equal Employment Opportunity Commission. They typically collect data on gender and race, and our research requires those really large data sets to draw patterns. They don’t ask for socioeconomic status or have an easy way to quantify that information. That’s not to say those are less important factors, or that no one is looking at them.
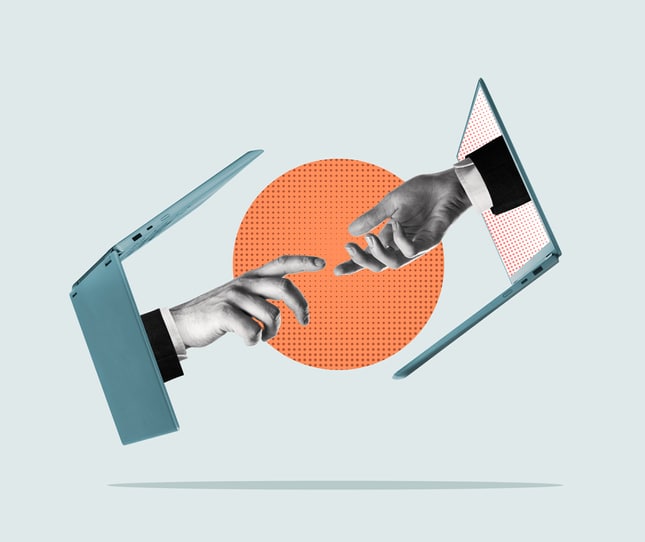
One of the papers you’re working on examines resumes written by self-identified men and women. It looks at how their resumes differ, and how that influenced their likelihood of being contacted for an interview.
So in this paper, we’re essentially looking at how men and women write their resumes differently and if that impacts hiring outcomes. Take resume screening algorithms, for example. One proposed way to reduce bias in these screening algorithms is to remove names from resumes to blind the applicant’s gender to the algorithm. But just removing names does very little, because there are so many other things that serve as proxies to someone’s gender. While our research is focused on people applying to jobs in the tech sector, this is true across occupations.
We find it’s easy to train an algorithm to accurately predict gender, even with names redacted.
Prasanna Parasurama
What are some of those gendered “tells” on a resume?
People write down hobbies and extracurricular activities, and some of those are very gendered. Dancing and ballet tend to denote female applicants; you’re more likely to see something like wrestling for male applicants.
Beyond hobbies, which is sort of obvious, is just how people write things, or the language they use. Female applicants tend to use a lot more affective words. Men, on the other hand, use more of what we call agentic words.
Can you explain that a little more?
In social psychology, social role theory argues that men are stereotyped to be more agentic, whereas women are stereotyped to be more communal, and that their communication styles reflect this. There’s essentially a list of agentic words that researchers have come up with that men use a lot more than women. And women are more likely to use affective words, like “warmly” or “closely,” which have to do with emotions or attitudes.
These communication differences between men and women have been demonstrated in social sciences before, which has helped inform our work. But we’re not just relying on social science tools—our conclusions are driven by our own data. If a word is able to predict that an applicant’s resume belongs to a female versus male applicant, then we assign different weights, depending on how accurately it can predict that. So we’re not just operating on theories.
Were there any gendered patterns that surprised you?
If you were to assign masculinity and femininity to particular words, an algorithm would likely assign “married” to be a feminine term in most contexts. But in this particular case, it’s actually more associated with men. Men are much more likely to use it in resumes, because it signals something different to society than when women use it.
One of the most predictive terms for men was references to parenthood. It’s much easier for men to reference kids than for women to reveal information about their household status. Women face a penalty where men receive a boost.
Prasanna Parasurama
Studies show that people perceive fathers as being more responsible employees, whereas mothers are regarded as less reliable in the workplace.
We haven’t studied this, but I would speculate that if you go on a platform like LinkedIn, men are more likely to disclose details about fatherhood, marriage, and kids than women are.
There were some other tidbits that I didn’t see coming, like the fact that women are much less likely to put their addresses on their resume.
Can AI predict race from a resume as easily as it can predict gender?
There’s surprisingly very little we know on that front. From existing literature outside of algorithmic literature, we know differences exist in terms of race, not just on the employer side, where there might be bias, but we also on the worker side. People of different races search for jobs differently. The question is, how do we take this into account in the algorithm? From a technical standpoint, it should be feasible to do the same thing we do with gender, but it just becomes a little bit harder to predict race in practice. The cues are so variable.
Gender is also more universal – no matter where you live, there are probably men and women and people who identify as in between or other. Whereas the concept of race can be very specific in different geographic regions. Racial identities in America are very different from racial identities in India, for instance. And in a place like India, religion matters a lot more than it does in the United States. So this conversation around algorithms and bias will look different across the globe.
Beyond screening resumes, how does AI impact people’s access to job opportunities?
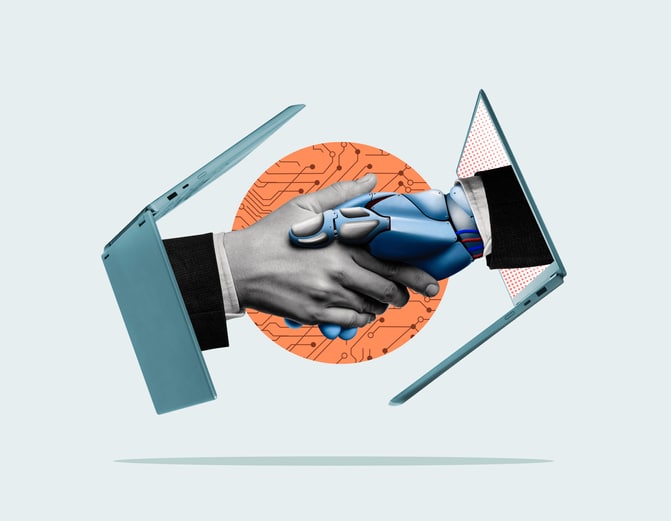
A lot of hiring platforms and labor market intermediaries such as LinkedIn use AI. Their task is to match workers to these different jobs. There’s so many jobs and so many workers. No one can manually go through each one. So they have to train algorithms based on existing behavior and existing design decisions on the platform to recommend applicants to particular jobs and vice versa. When we talk about algorithmic hiring, it’s not just hiring per se, but spaces like these which dictate what opportunities you’re exposed to. It has a huge impact on who ends up with what job.
What impact do you want your research to have in the real world? Do you think that we actually should use algorithms to figure out gender or race? Is it even possible to blind AI to gender or race?
Algorithms are here to stay, for better or worse. We need them. When we think about algorithmic hiring, I think people picture an actual robot deciding who to hire. That’s not the case. Algorithms are typically only taking the space of the initial part of hiring.
I think overall, algorithms make our lives better. They can recommend a job to you based on more sophisticated factors than when the job was chronologically posted. There’s also no reason to believe that a human will be less biased than an algorithm.
Prasanna Parasurama
I think the consensus is that we can’t blind the algorithm to gender or other factors. Instead, we do have to take people’s demographics into account and monitor outcomes to correct for any sort of demonstrable bias.
LinkedIn, for example, does a fairly good job publishing research on how they train their algorithms. It’s better to address the problem head on, to take demographic factors into account upfront and make sure that there aren’t drastic differences in outcomes between different demographics.
What advice would you give to hopeful job candidates navigating these systems?
Years of research have shown that going through a connection or a referral is by far the best way to increase your odds of getting an interview—by a factor of literally 200 to 300 percent. Hiring is still a very personal thing. People typically trust people they know.