When you’re browsing the internet for something to buy, watch, listen to, or rent, chances are that you will scan online recommendations before you make your purchase. It makes sense. With an overabundance of options in front of you, it can be difficult to know exactly which movie or garment or holiday gift is the best fit.
Personalized recommendation systems help users navigate the often-confusing labyrinth of online content. They take a lot of the legwork out of decision-making. And they are an increasingly commonplace function of our online behavior. All of which is in your best interest as a consumer, right?
Yes and no, said Jesse Bockstedt, associate professor of information systems and operations management at Emory’s Goizueta Business School. Bockstedt has produced a body of research in recent years that reveals a number of issues with recommendation systems that should be on the radar of organizations and users alike.
While user ratings, often shown as stars on a five- or ten-point scale, can help you decide whether or not to go ahead and make a selection, online recommendations can also create a bias towards a product or experience that might have little or nothing to do with your actual preferences, Bockstedt said. Simply put, you’re more likely to watch, listen to, or buy something because it’s been recommended. And not only that. When it comes to recommending the thing you’ve just watched, listened to, or bought yourself, your own rating might also be heavily influenced by the way it was recommended to you in the first place.
“Our research has shown that when a consumer is presented with a product recommendation that has a predicted preference rating—for example, we think you’ll like this movie or it has four and a half out of five stars—this information creates a bias in their preferences,” Bockstedt said. “The user will report liking the item more after they consume it if the system’s initial recommendation was high, and they say they like it less post-consumption, if the system’s recommendation was low. This holds even if the system recommendations are completely made up and random. So the information presented to the user in the recommendation creates a bias in how they perceive the item even after they’ve actually consumed or used it.”
This in turn creates a feedback loop which can reflect authentic preference, but this preference is very likely to be contaminated by bias. And that’s a problem, Bockstedt said.
“Once you have error baked into your recommendation system via this biased feedback loop, it’s going to reproduce and reproduce so that as an organization you’re pushing your customers towards certain types of products or content and not others—albeit unintentionally,” Bockstedt explained. “And for users or consumers, it’s also problematic in the sense that you’re taking the recommendations at face value, trusting them to be accurate while in fact they may not be. So there’s a trust issue right there.”
Online recommendation systems can also potentially open the door to less than scrupulous behaviors, Bockstedt added.
Because ratings can anchor user preferences and choices to one product over another, who’s to say organizations might not actually leverage the effect to promote more expensive options to their users? In other words, systems have the potential to be manipulated such that customers pay more—and pay more for something that they may not in fact have chosen in the first place.
Addressing recommendation system-induced bias is imperative, Bockstedt said, because these systems are essentially here to stay. So how do you go about attenuating the effect?
His latest paper sheds new and critical light on this. Together with Gediminas Adomavicius and Shawn P. Curley of the University of Minnesota and Indiana University’s Jingjing Zhang, Bockstedt ran a series of lab experiments to determine whether user bias could be eliminated or mitigated by showing users different types of recommendations or rating systems. Specifically they wanted to see if different formats or interface displays could diminish the bias effect on users. And what they found is highly significant.
“When you show users a rating or recommendation that is highly focused on a specific numerical value—a percentage or a mark out of ten, say—we found that the bias effect is particularly pronounced. Consumers will experience this preference bias more strongly if the recommendation is presented with a clear numerical value.”
But the bias effect is significantly less when the rating display is less numerical and more graphic, Bockstedt noted. “We found that by taking out the numbers or making them less specific, the bias effect was considerably reduced. Put it this way, show them a precise number or percentage and the bias becomes stronger. Take away the number or make it more graphic—a star system without a percentage or a thumbs up or down, for instance—and you immediately see considerably less bias in their preferences.”
To reach these findings, Bockstedt and his co-authors ran a series of randomized lab experiments with volunteers. The experiments variously had participants complete tasks. These included liking (or disliking) a number of jokes that had been recommended to them in different ways, and then being asked to rate the jokes themselves, again using a range of techniques to manipulate their responses by exposing them to system ratings.
In one experiment, for instance, participants were told that “the system” predicted they would love or hate a joke. Here they were given this information two ways: one was a graphic interface with “hate it” and “love it” at either end of a scale and the other a numerical interface with a score out of five.
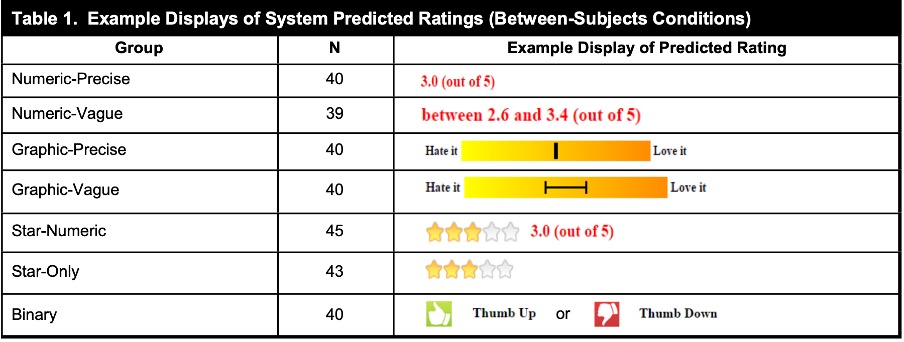
The results were conclusive across the studies. When participants were shown something graphic they experienced less preference bias. When they were shown a concrete number, they were more biased or anchored in their preference. Interestingly, Bockstedt and his co-authors also found that when users were asked to rate something using the same display or interface as they had seen before experiencing the product, the bias effect was more acute.
“This is called scale compatibility, and it has been shown to be an important factor in anchoring and adjustment,” Bockstedt said. “Specifically, if you show someone a specific rating display—let’s say a percentage or a score out of five—and then you ask them to rate the thing using the exact same display or format, you’re going to see even more bias in their response. And the opposite is true. So if you show them a percentage first, then ask them to rate it in nonnumerical terms—they either loved it or hated it, say—the bias can be reduced.”
The implications of these findings should be food for thought for organizations that use online recommendations systems, Bockstedt added. Reducing bias in ratings should very much be front of mind for companies looking to drive excellent user experience, customer satisfaction, and loyalty in an online market that is becoming more crowded and competitive.
“We’ve seen some of the big players like Netflix, Rotten Tomatoes, Macy’s, and others take more proactive measures over time and adopt more sophisticated ratings systems that do more to reduce bias,” he said. “Other players would do well to pay close heed to this.”
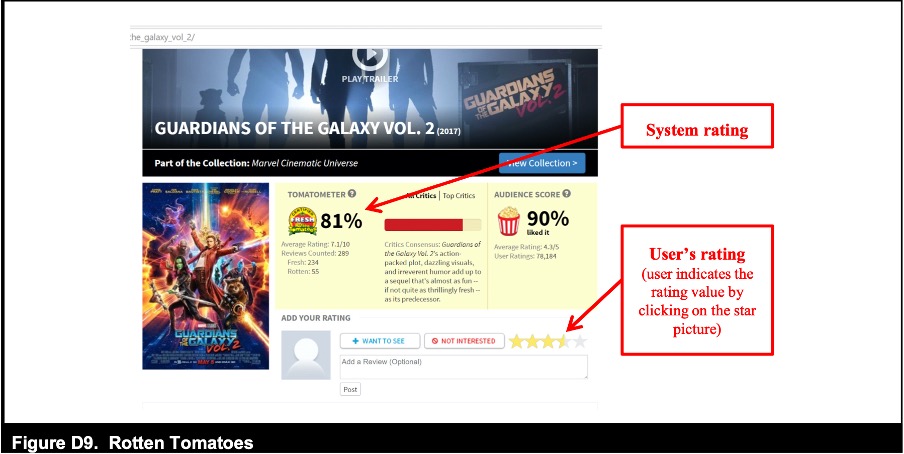